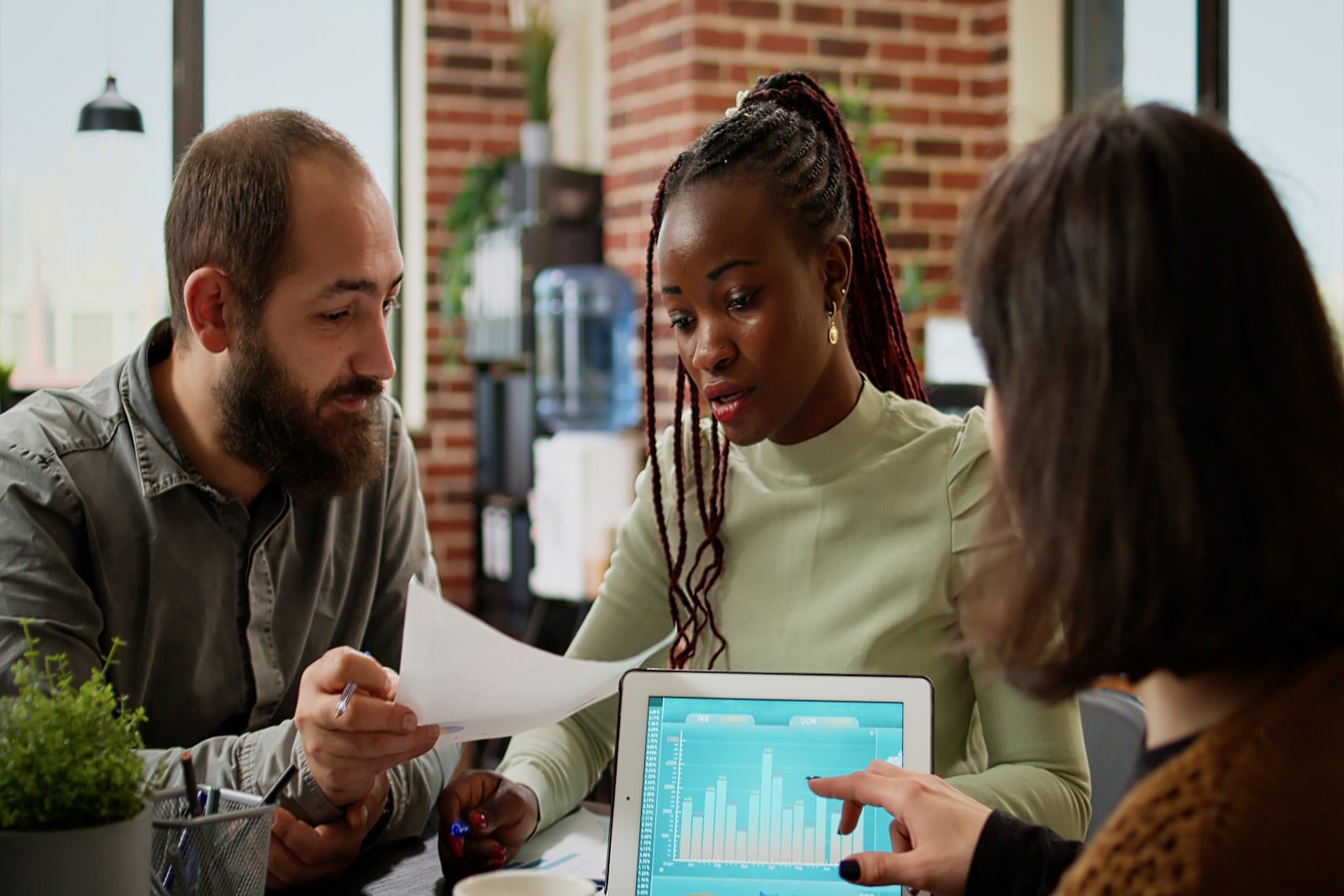
Analytics in Learner Management Systems
In recent years, educational institutions and organisations have increasingly turned to data to enhance learner outcomes. The integration of advanced analytics in learner management systems (LMS) has revolutionised how educators assess and support students. By harnessing data-driven insights, these systems are not only providing a clearer picture of learner progress but are also enabling proactive interventions that predict success and identify potential challenges before they become critical.
Introduction to Advanced Analytics in LMS
Advanced analytics in a learner management system refers to the use of sophisticated techniques to extract valuable insights from the vast amounts of data generated by learners’ interactions within the system. These analytics go beyond simple reporting and dashboards, utilising complex algorithms and statistical models to uncover patterns that can help predict learner outcomes.
Educational institutions and organisations now have the ability to track and analyse a learner’s journey, providing an invaluable understanding of their behaviour, engagement, and progress.
Data Types Collected by LMS for Analytics
A learner management system collects various types of data that can be used to evaluate and predict a learner’s performance and success. Here are the primary data types that play a crucial role in advanced analytics:
- Learner Engagement Metrics: These metrics track how actively students are engaging with the course material. It includes data on how often learners log in, the amount of time spent on each module, and their interaction with various learning materials like videos, quizzes, and discussion forums. Engagement data is one of the most telling indicators of a student’s commitment to their learning journey.
- Assessment Performance: The scores and completion rates of quizzes, assignments, and exams offer a direct insight into a learner’s understanding of the subject matter. A learner’s ability to consistently perform well in assessments is often a strong predictor of success in the course.
- Learning Behaviour Patterns: Learners’ navigation through the course provides valuable insights into their learning preferences. For instance, if a learner frequently revisits certain sections, it may indicate areas where they are struggling, whereas those who explore additional resources may be demonstrating a proactive approach to learning.
- Demographic Data: Information such as the learner’s age, background, and prior educational experiences can help tailor the learning experience. This data can be especially useful when combined with other metrics to personalise recommendations and interventions.
Predictive Models for Learner Success
One of the most powerful features of advanced analytics in a learner management system is the ability to predict future learner success or failure. Predictive analytics involves using historical data to identify patterns that can forecast future outcomes.
- Regression Analysis: By examining the relationship between different variables, such as engagement levels and assessment performance, regression analysis can predict the likelihood of a learner succeeding or struggling in the course.
- Machine Learning Models: These models, such as decision trees and neural networks, use large datasets to recognise complex patterns in learner behaviour. By training these models on past data, they can identify which factors are most strongly correlated with success or failure, allowing for more accurate predictions.
- Clustering: This technique groups learners with similar characteristics together, enabling educators to identify commonalities in behaviour and performance. It helps personalise support strategies for different learner segments, ensuring that each learner receives the right interventions.
Predicting Dropout Rates
Dropout rates remain a significant concern for many educational institutions and organisations.
Common reasons for learner dropout include lack of engagement, personal challenges, and misunderstandings about course requirements. Advanced analytics can track warning signs in real time, enabling early intervention.
- Decreased Logins: A significant reduction in login frequency often signals a lack of interest or motivation. This is one of the earliest indicators that a learner may be at risk of disengaging.
- Unsubmitted Assignments: If a learner consistently fails to submit assignments, it could be a sign that they are struggling with the content or experiencing personal challenges that prevent them from staying on track.
- Lack of Forum Participation: Low engagement in discussion forums or group activities can also be a strong indicator that a learner is no longer engaged with the course.
By monitoring these indicators, a learner management system can flag at-risk students and provide timely alerts to instructors. This allows educators to step in early, offering additional support or even adapting the course materials to better suit the learner’s needs.
Real-Time Monitoring and Interventions
One of the most powerful aspects of analytics in a learner management system is its ability to enable real-time monitoring and interventions. As learners progress through a course, their data is continuously analysed, providing up-to-date insights into their engagement and performance.
- Continuous Monitoring: LMS administrators and instructors can track learner progress through dashboards that display key metrics, such as assessment scores, activity logs, and engagement levels. This allows them to quickly identify students who may be struggling and offer immediate support.
- Adaptive Learning Technologies: Many LMS platforms incorporate adaptive learning technologies that adjust the course material in real time based on learner progress. For example, if a learner is struggling with a particular topic, the system can provide additional resources or adjust the difficulty level to help them succeed.
- Personalised Recommendations: Based on the data collected, the learner management system can provide personalised recommendations to students, such as suggesting additional reading materials, revisiting certain modules, or offering extra practice exercises.
Conclusion
The integration of advanced analytics into learner management systems is transforming the way educational institutions and organisations manage learner progress.
At Sound Idea Digital, we specialise in implementing and optimising learner management systems that leverage the power of analytics to support learner success. If you are looking to enhance your organisation’s approach to learner progress and retention, get in touch with us today to discover how we can help.